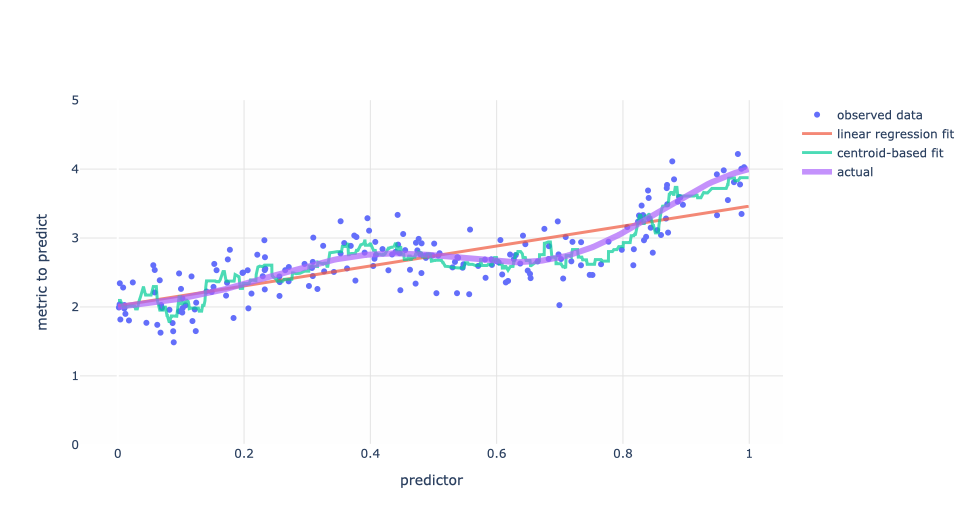
There are many ways to forecast business metrics. Both qualitative and quantitative approaches have their benefits and costs and work under different conditions. The two most important things anyone can do when going down the forecast route is to (a) understand the limitations of each approach and (b) check one’s own preferences.
In this article, I give an overview of three types of approaches and behaviors for forecasting. For a larger treatment, connect with me and let’s work together.
WISHFUL FORECASTING
“Forecast by ostrich” is usually a strategy employed when starting something new. It’s neither based on experience nor gut nor even some data point. This is hope and a prayer. Sometimes though, faith in something is what’s needed to get things off the ground, just don’t drink this kool-aid if success visits you.
“Forecast by groundhog” never works, but superstition is a hell of a drug and humans are notorious for finding patterns / correlation where none exist. Forecast-by-groundhog is usually the method of choice when data is limited. But a good way to use this method is to have it be a baseline against all other methods. If you can’t beat the groundhog, then you have a contrarian signal or another groundhog. Don’t create a cult around the groundhog. The groundhog doesn’t actually predict the weather, Phil Connors does who, in theory, uses weather prediction models based on physics and data (which can go wrong sometimes!).
SOFT FORECASTING
“Forecast by experience” works directionally and when the underlying business / economic conditions are generally stable. The downside is reputational harm to you when you no longer have a pulse on the environment. March 2020 broke this approach, but businesses went along with it anyway because “behaviors changed so much historical data couldn’t be used reliably”. To some extent this was true since comparison of year over year growth was senseless. However, if the underlying behaviors have changed so much, then we should also be careful about our experience.
“Forecast by echo chamber / peer pressure / social contagion” is a common soft forecasting practice wherein forward-looking statements are more a result of external data and opinion than one’s own business analytics or even one’s own experience. There are perils especially in times of high uncertainty when one or two entities make public, forward-looking statements that could be aggressive. It’s hard to come in with a statement that is different from this influential group since the game theory is usually not in one’s favor to challenge. As a consequence, we get a coalescence around the benchmark makers and the formation of an echo chamber — think 2020 where every “pandemic company” was forecasting massive sustained growth and now we have the steady parade of layoffs.
I am being a bit harsh because of the benefit of hindsight with respect to 2020 forecasts. But we can examine the layoff wave. Perhaps there really are systemic economic concerns, but we may also be creating a self-fulfilling prophecy. As always, there are times when the crowd is right and independent evaluations of the data will come to the same conclusion. At the same time, the crowd doesn’t know about your particular situation. What may be true in a macro sense may be untrue for you. You have to know if you are simply following the leader or doing your own due diligence and coming to similar conclusions for your use case.
Forecast by eagle-eye chart reading: We’ve all done it. We’ve looked at a chart or three, drawn a trend line over the data and called it a night. This is probably a reliable enough method, directionally, if the trends are “obvious” and we’re not making mission critical decisions. The fundamental problem is that there is no deeper insight into the underlying factors that move the metrics of interest.
THE DRAGONS OF FORECASTING WITH DATA
Forecasting with data is an entire academic discipline that encompasses topics like linear and non-linear regression, time series analysis, cohort and waterfall analysis, clustering, and so on.
In a nutshell, the dragons of forecasting with data are: garbage in / garbage out, expectations, and weaponization.
Garbage In / Garbage Out
Garbage in is poor data [missing, imprecise, erroneous, insufficient] and poor methodology [just because there’s a command in a software package called “forecast” does’t mean it will divine your intentions]. Too often, the convenience of saying “we have data therefore we have insights” is never challenged. And too often have I heard “we just need a forecast, the data doesn’t have to be perfect”.
The latter is a fallacy of sorts. Anyone working with data has no expectations of perfect data, but there is a maximal tolerance of how bad data can be after which it is unusable. The former is a misunderstanding of the analytics funnel and process. Data is needed for insights, but data doesn’t guarantee it.
Garbage out is poor interpretation, which results in poor decision-making. One example of poor model interpretation is not understanding the implicit uncertainty in the data. Most models spit back an average, but confidence intervals, credible intervals, prediction intervals or some range to convey variance are helpful.
Common refrains you’ll hear from me are “not everything has to be metricized”, “data doesn’t capture everything all the time”, or “MATH: minimal analysis that helps” (an acronym I made up many years ago that I’ll write more about in the coming weeks). But don’t interpet that to mean “since data doesn’t capture everything, let’s just make stuff up”. Measure what makes sense to be measured. Don’t overcomplicate (to whose standards?) stuff, but don’t shy away from second order insights by saying “it’s too complicated”. You’re not making simple decisions!
Expectation
The dragon of “expectation” is that there is a cultural asymmetry for tolerating imprecise results when working with data vs when not. For whatever reason, we, as a society, tend to be ok with the wild assumptions and potentially disastrous outcomes from the finger-in-the-wind approaches given above, but the moment we know that “math” was involved, the expectations go from “directional” to “crystal ball” in a hurry. This happens everywhere. Big companies, small companies, big budgets, small budgets.
Quantitative forecasting is not a crystal ball. Rather it’s a numerical guide so that everyone involved in decision making is, at least, working within the same context. “I think we’ll see good growth if we do X” is a vague and useless statement since “good” is subject to everyone’s interpretation and risk tolerance. The worst (and most common) thing that happens is we all assume that everyone else’s interpretation is equivalent to our own. Rather, having a discussion around “I think we’ll see 15-20% growth if we do X” focuses the conversation on the 15-20%, the X, and if that’s sufficient. Maybe we don’t want to take on the cost of X for only 15-20%. Maybe 15-20% is unbelievable.
Weaponization
The dragon of “weaponization” is when we use forecasting (or data more broadly) in circular ways. The overarching agreement should be to arrive at a minimally subjective truth (note I’m not saying “objective” — a discussion for another day). “I need for the numbers to show X” is inherently a corruption of forecasting / data practices.
Another form of weaponization is cherry picking pseudo-correlative factors to show the a priori desired effect.
Both forms of weaponization have us wading into political waters where there are more than just dragons present.
Reaching Out
If you are in need for business forecasting or want to set up the practice in your organization, connect with me and let’s work together!