If there is no data, then there can’t be any insights. That seems to be tautologically true. We can splice the word “data” to mean many things and formats, but at its most abstract, data is “information”. So if we don’t have information, we can’t have insights.
The contrapositive here is that “if we have insights, then we must have had information (data)”.
But! As is the ol’ “correlation does not imply causation” mantra, we have “If we have information (data), it does not imply insights”. The only things that can be guaranteed with data are data organization, data architecture, and data analytics. Insights are the result of the storytelling from analytics. Yet, so often with data practices (data analytics, data science, for examples), the guarantee of the existence of insights because of the existence of data seems to never be questioned.
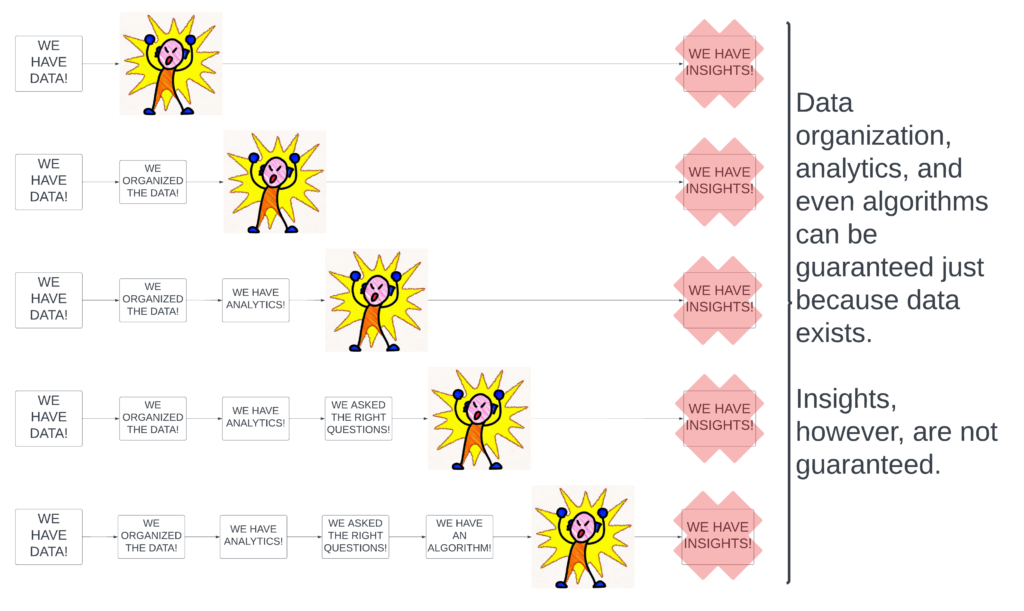
More dangerously, the belief that insights are guaranteed is held almost axiomatically within leadership teams to the detriment of the productivity of their business analytics and product teams.
A generic question and answer template goes like this regardless of the business unit: “We’re seeing a drop in <insert KPI that should be going up>, can we find out why? Let’s slice our customer data by geo, tenure, SKU, product activity, help desk outreach, etc. and see which cross sections are the most problematic and address those.”
This is standard operating procedure for business analytics. One of the axioms being that “our large store of data is sufficient to provide (business) insights and for us to take action”. Another axiom is that there is a single root cause. Both of these axioms are falsifiable not just in the strict logical sense of “there exists a counterexample”, but even in the looser, social sense of “this mode of thinking is more right than wrong”, which it is not.
While any business operator, myself included, who has used data to make informed decisions can give plenty of anecdotes about the success and existence of the data-to-insights pipeline, the main problem lies in its self-fulfilling prophecy. Decision makers simply choose to forget those moments when they “simply made a decision” even when there was no clear data signal explaining the metric movement.
An existing analytics practice identified a not-so-pleasant movement in a key metric. A decision has to be madeĀ somehow to cure the problematic metric. A set of actions to take to treat the problematic metric is inferred through a reductive analysis process. With enough data cuts, some behavior somewhere will align with the metric under question and that behavior is deemed the culprit. This is the business analytics equivalent of p-hacking. Usually, some intuitive treatments (read: “veteran experience” or worse coming from “thought leaders”) are prescribed and it’s a wish and a prayer if that will fix things. On the off chance that some correlative behavior hasn’t been found, the response from decision makers is “to dig further” until there is an explanation.
Better business analytics practices would be to
- incorporate statistical reasoning accounting for the implicit, multiple, correlated hypotheses being tested (in practice, I have yet to see a biz ops / fin ops team run statistical tests)
- maintain an openness to the possibility of multiple, simultaneous, causal effects
- maintain an openness that the data cannot give a signal
- make a commitment to a standard practice of having a control group always (e.g., A/B test) so that if / when a metric does go off balance, there is a baseline to help determine if the factors are macroeconomic or limited to changes made in business practices
- create metrics at every chain of the data funnel so that metrics can actually roll up to the global level in a mathematically accurate way
- differentiate between how the metric moves mathematically vs how the metric moves as a consequence of behavior
- develop and maintain a business simulator — I will go so far as to say if a business can’t simulate itself it doesn’t have a handle on how its processes generate value and impact its KPIs (that’s not to say that a business can’t be successful even if it can’t map its actions to its metrics (an overwhelming number of businesses are like this!), but it is to say, that generating insights becomes harder)
Too many business analytics practices focus on spinning up endless, useless dashboards which only give bare bones “alerts” with nary a sense of a true positive rate (i.e., an alert was triggered and it was real).
Reach out if you want to take your Business Analytics practice to 11.